Data Preparation and Data Quality
Data-driven systems and machine learning-based decisions are becoming increasingly important and are having an impact on our everyday lives. The prerequisite for this is good data quality, which must be ensured by data preparation.
Keywords:
|
Data Preparation
Data Quality
Data Cleaning
Data Wrangling
Evaluation
|
GouDa: Generation of universal Data Sets
GouDa is a tool for the generation of universal data sets to evaluate and compare existing data preparation tools and new research approaches. It supports diverse error types and arbitrary error rates. Ground truth is provided as well. It thus permits better analysis and evaluation of data preparation pipelines and simplifies the reproducibility of results.
|
- Diverse error types - Arbitrary error rates - Ground truth provided - Scalable - Publicly available: Zenodo GitLab |
Publication: V. Restat, G. Boerner, A. Conrad, U. Störl: GouDa - Generation of universal Data Sets, DEEM@SIGMOD 2022, https://doi.org/10.1145/3533028.3533311
Presentation GouDa DEEM@SIGMOD 2022
Holistic Data Preparation Tool
We propose the design of a holistic tool to support domain experts in data preparation:
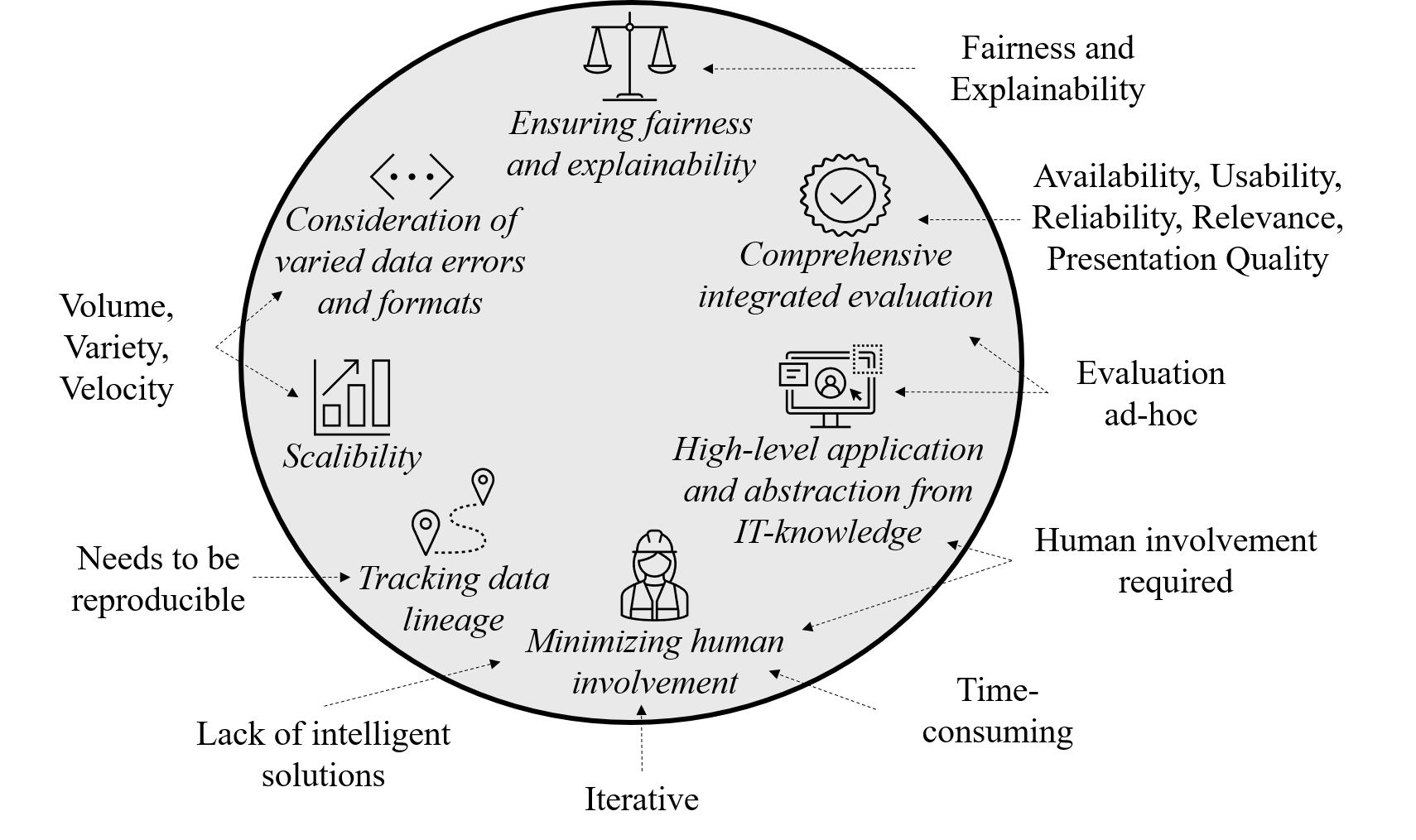
Publication: V. Restat, M. Klettke, U. Störl: Towards a Holistic Data Preparation Tool, DataPlat@EDBT 2022, https://ceur-ws.org/Vol-3135/dataplat_short1.pdf
Publication: V. Restat: Towards “all-inclusive” Data Preparation to ensure Data Quality, arXiv:2308.14617
CheDDaR: Checking Data - Data Quality Review
Data-driven systems and machine learning-based decisions are becoming increasingly important and are having an impact on our everyday lives. The prerequisite for good results and decisions is good data quality, which must be ensured by preprocessing the data. Therefore, we propose CheDDaR - a framework of metrics that allows for a flexible evaluation of data quality and data preparation results.
|
- Detailed evaluation of data quality - Comparison of data sets - Considers time of evaluation - Can be used flexibly |
Publication: V. Restat, M. Klettke, U. Störl: “FAIR” is not enough – A Metrics Framework to ensure Data Quality through Data Preparation, DE4DS@BTW 2023, https://doi.org/10.18420/BTW2023-61
Poster
I. Diestelkämper, R. Diestelkämper, and V. Restat: CheDDaR: Checking Data – Data Quality Report, BTW 2025
FONDUE: Fine-tuned Optimization: Nurturing Data Usability & Efficiency
FONDUE is a well-founded end-to-end data quality optimizer. In contrast to many studies that consider data cleaning in the context of machine learning, our approach focuses on areas of application where the downstream analysis is unknown. Our proposed adaptive and easily extendable framework operates similarly to proven methods of database query optimization. Analogously, it consists of the following parts: Rule-based optimization, where the appropriate data cleaning algorithms are selected based on use case constraints, optimizer hints in the form of best practices, and cost-based optimization, where the cost is measured in terms of data quality. Accordingly, the result is a data cleaning pipeline that provides the best possible data quality. The choice of different optimization goals enables further flexibility, e.g. for environments with limited resources.
|
- End-to-end data quality optimizer - Different optimization goals - Independent of the subsequent analysis - Adaptive and flexible |
Publication: V. Restat, M. Klettke, U. Störl: Towards an End-to-End Data Quality Optimizer, DataPlat@ICDE 2024, https://doi.org/10.1109/ICDEW61823.2024.00039
Publication: V. Restat, I. Diestelkämper, M. Klettke, U. Störl: FONDUE - Fine-tuned Optimization: Nurturing Data Usability & Efficiency, Journal of Big Data, 2025 https://doi.org/10.1186/s40537-025-01158-x
ALPINE: Abstract Language for Pipeline Integration and Execution
When working with data, it is essential to ensure data quality and clean data of errors. This is usually done with a data cleaning pipeline. The execution of such a pipeline is possible with a variety of tools. However, to increase reusability, there should be a way to describe pipelines independently of technology. So far, there is no such technology-independent description. We therefore present ALPINE, a language for describing data cleaning pipelines. This abstracts from the concrete implementation.
|
- Technology-independent description - Enables implementation and execution in various technologies - Promotes reusability - Exchange format between different systems |
Publication: V. Restat, U. Störl: ALPINE: Abstract Language for Pipeline Integration and Execution, DE4DS@BTW 2025
Student theses
We regularly publish new topics for theses. An overview of open topics can be found here: dbis theses
Assigned
- Reihenfolge von Data Cleaning Pipelines (praktischer Fokus)
- Datenqualität von semi-strukturierten Daten
Ongoing
- Finden optimaler Data Cleaning Pipelines mittels kostenbasierter Optimierung
- Reihenfolge von Data Cleaning Pipelines (theoretischer Fokus)
Overview (live generated gantt chart)
Completed
- Testdatengenerierung für die Analyse von Data Preparation Pipelines - G. Boerner (Bachelor)
- Analyse von Data Cleaning Tools - L. Lafleur (Bachelor)
- Analyse von Data Cleaning Pipelines - O. Schwammberger (Bachelor)
- Data Cleaning in Data Streaming Pipelines - N. Rodenhausen (Master)
- Reproduzierbarkeit von Data Cleaning Pipelines - A. Schwarz (Master)
- Analyse von Missing Value Imputation - K. Tejkl (Master)
- Visualisierung von Missing Values - D. Giesen (Bachelor)
- Reproducibility in Data Preprocessing: An Evaluation of Open Source Tools - S. Grimm (Bachelor)
- Data-Streaming-Technologien für Data Cleaning - C. Antonin (Bachelor)
- Data preparation of semi-structured data - A. Zeidler (Master)
- Fairness in Data Preprocessing - M. Werner (Bachelor)
- Constraints for Missing Value Imputation - A. Herling (Bachelor)
- Trade-offs between Performance-oriented and Sustainability-oriented Approaches for MLWorkloads in the Cloud - D. Senzel (Master)
- CheDDaR: Konzeption und prototypische Implementierung eines Tools zur Analyse von Datenqualität - I. Diestelkämper (Master)
- Data-Streaming: Technologie-Studien im Vergleich - F. Meier (Bachelor)